Zhiliu Yang, a Ph.D. student in electrical and computer engineering at Clarkson University, along with his advisor, Dr. Chen Liu, associate professor of electrical and computer engineering recently presented their newly published paper “pi-Map: A Decision-Based Sensor Fusion with Global Optimization for Indoor Mapping” at the 2020 IEEE/RSJ International Conference on Intelligent Robots and Systems (IROS 2020). The conference is being held on demand and virtually from October 25 through November 25 this year.
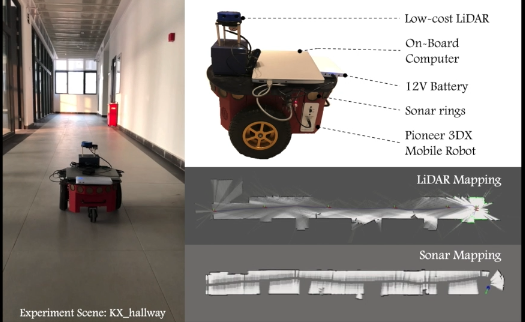
The IEEE/RSJ International Conference on Intelligent Robots and Systems (IROS) is one of the largest and most impacting robotics research conferences worldwide. Established in 1988 and held annually, IROS provides an international forum for the international robotics research community to explore the frontier of science and technology in intelligent robots and smart machines.
Indoor robots are widely used across many aspects of our daily lives, from fetching products in Amazon warehouses, delivering food and beverage in malls and restaurants, to vacuuming and cleaning in our own homes. However, the universal deployment of indoor autonomous robots can only be enabled with the availability of affordable, reliable, and scalable indoor maps. LiDAR sensors, though very popular in indoor mapping, cannot be used to generate precise indoor maps due to their inability to handle reflective objects, such as glass doors and French windows. Similarly, sonar sensors have been used to construct indoor maps as well. But sonar-based maps suffer from inaccuracy caused by sonar cross-talk, corner effects, large noise, etc. Nonetheless, sonar sensors can handle glass and reflective objects quite well, which perfectly complements LiDAR sensors.
Yang and Liu’s paper proposes a different way to map, called pi-map, which is a tightly coupled fusion mechanism that dynamically consumes LiDAR and sonar data to generate reliable and scalable indoor maps for autonomous robot navigation. It works in three stages: the first LiDAR scan matching stage efficiently generates initial key localization poses; the second optimization stage is used to eliminate errors accumulated from the previous stage and guarantees that accurate large-scale maps can be generated; then the final revisit scan fusion stage effectively fuses the LiDAR map and the sonar map to generate a highly accurate representation of the indoor environment. They have evaluated pi-map in both large and small environments and can verify its superiority over existing fusion methods.
Click here for a video demonstration: https://www.youtube.com/watch?v=8q2F21gky5o&feature=youtu.be