Zander Blasingame, a Ph.D. student in the electrical and computer engineering department at Clarkson University, along with his advisor, Dr. Chen Liu, an associate professor of electrical and computer engineering, presented their recently published paper titled “Leveraging Adversarial Learning for the Detection of Morphing Attacks” at International Joint Conference on Biometrics (IJCB 2021) held virtually August 4-7.
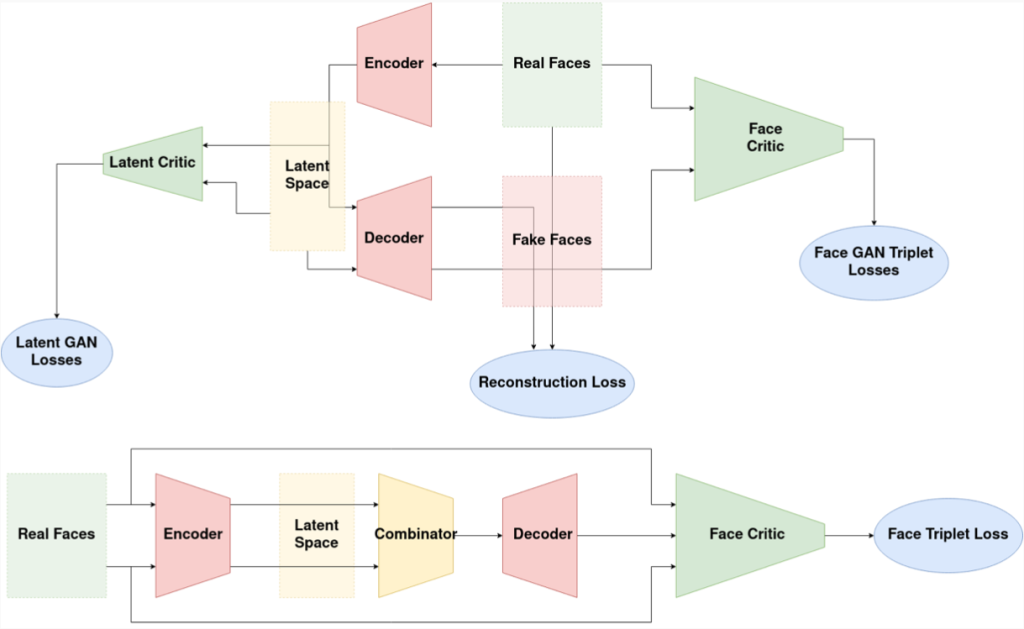
The 2021 International Joint Conference on Biometrics (IJCB 2021) combines two major biometrics conferences, IEEE Biometrics Theory, Applications, and Systems (BTAS) Conference and the International Conference on Biometrics (ICB). The IJCB is one of the top conferences for Biometrics and is visited by countless internationally recognized Biometrics researchers.
Face recognition systems (FRS) have become ever more omnipresent in an increasing number of tasks associated with our daily lives. They have been used as one of the common biometric modalities for identity verification in applications as common as unlocking smartphones, to facility access control, banking, e-commerce, as well as law enforcement. Unfortunately, while FRS have been shown to reach low false rejection and acceptance rates, they are especially vulnerable to a specific type of emerging attacks, known as face morphing attack. The morphing attack targets the breakdown of a fundamental property of biometric security, i.e., the one-to-one mapping from an individual’s biometric data to his/her identity. This is achieved by creating a morphed face containing the biometric data of two identities in such a manner that this one image triggers a match with two disjoint identities, violating the fundamental principle.
Many of the existing morphing attack detection (MAD) approaches have been trained and evaluated on datasets with a limited variation of image characteristics, which can make the approach prone to overfitting. Additionally, there has been difficulty in developing MAD algorithms which can generalize beyond the morphing attack they were trained on, as shown by the most recent NIST FRVT MORPH report. Furthermore, the Single image based MAD (S-MAD) problem has had poor performance, especially when compared to its counterpart, Differential based MAD (D-MAD).
In this work, Blasingame and Liu propose a novel architecture for training deep learning-based S-MAD algorithms that leverages adversarial learning to train a more robust detector. The performance of the proposed S-MAD method is benchmarked against the state-of-the-art S-MAD algorithm. The proposed method has demonstrated superior and robust detection performance when evaluated against different morphing attacks.
Blasingame graduated from Clarkson with a bachelor of science degree in Computer Engineering in 2018.
This research is funded as a special project from the Department of Homeland Security via Clarkson’s Center for Identification Technology Research (CITeR).