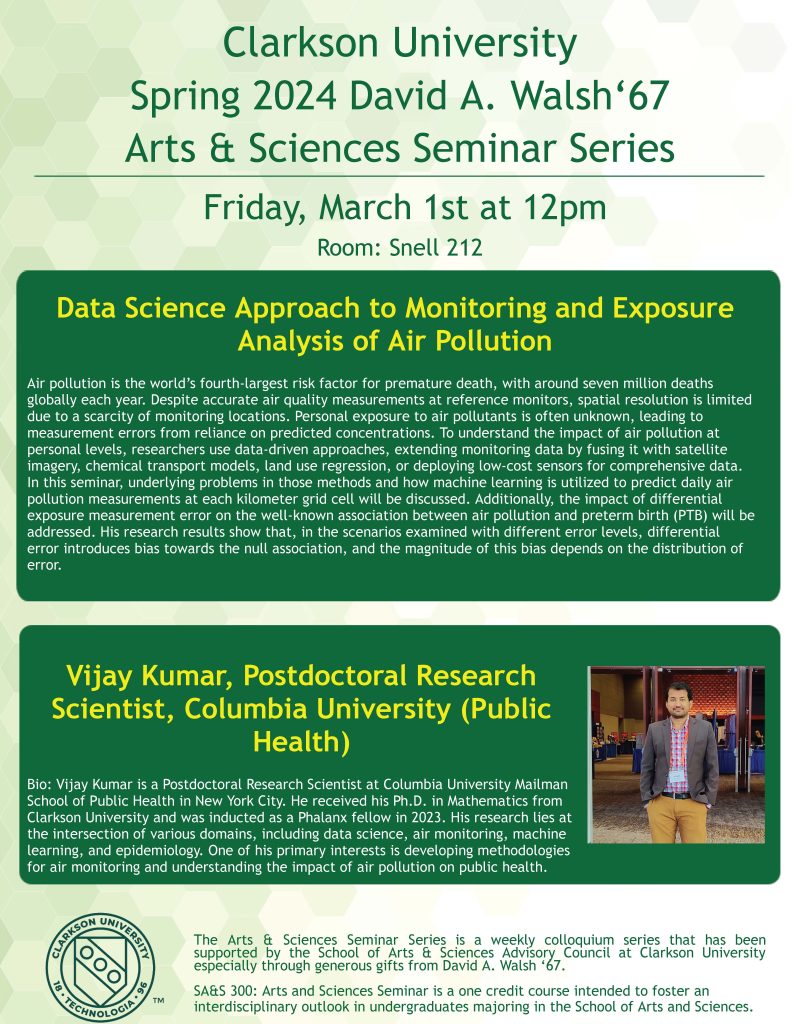
David A Walsh Arts and Science Seminar talk this Friday March 1st at noon in Snell 212.
Vijay Kumar, Postdoctoral Research Scientist at Columbia University Mailman School of Public Health in New York City, will speak on “Data Science Approach to Monitoring and Exposure Analysis of Air Pollution”.
Bio: Vijay Kumar is a Postdoctoral Research Scientist at Columbia University Mailman School of Public Health in New York City. He received his Ph.D. in Mathematics from Clarkson University and was inducted as a Phalanx fellow in 2023. His research lies at the intersection of various domains, including data science, air monitoring, machine learning, and epidemiology. One of his primary interests is developing methodologies for air monitoring and understanding the impact of air pollution on public health.
Abstract:
Air pollution is the world’s fourth-largest risk factor for premature death, with around seven million deaths globally each year. Despite accurate air quality measurements at reference monitors, spatial resolution is limited due to a scarcity of monitoring locations. Personal exposure to air pollutants is often unknown, leading to measurement errors from reliance on predicted concentrations. To understand the impact of air pollution at personal levels,
researchers use data-driven approaches, extending monitoring data by fusing it with satellite imagery, chemical transport models, land use regression, or deploying low-cost sensors for comprehensive data. In this seminar, underlying problems in those methods and how machine learning is utilized to predict daily air pollution measurements at each kilometer grid cell will be
discussed. Additionally, the impact of differential exposure measurement error on the well-known association between air pollution and preterm birth (PTB) will be addressed. His research results show that, in the scenarios examined with different error levels, differential error introduces bias towards the null association, and the magnitude of this bias depends on the distribution of error.